ONGOING AND PAST PROJECTS
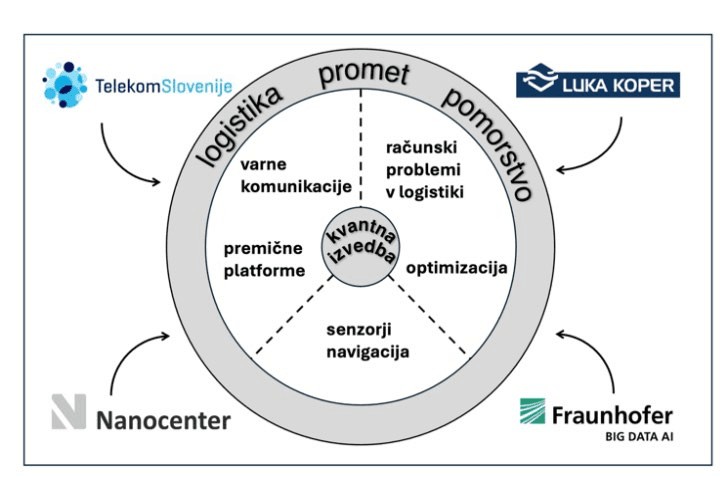
KTTK21: consortium -Ongoing
The project explores quantum technologies for transportation, communication, and logistics in Slovenia, leveraging the country’s strategic position and research expertise. It focuses on integrating quantum and classical methods to address challenges like vehicle routing, secure communications, and satellite-independent navigation. Key goals include developing quantum reservoir computing, secure quantum communication networks, advanced quantum sensors, and hybrid optimization algorithms. Practical applications target logistics optimization, robust communication for moving objects, and precision navigation. The project combines theory, experiments, and simulations while fostering interdisciplinary collaboration and innovation.
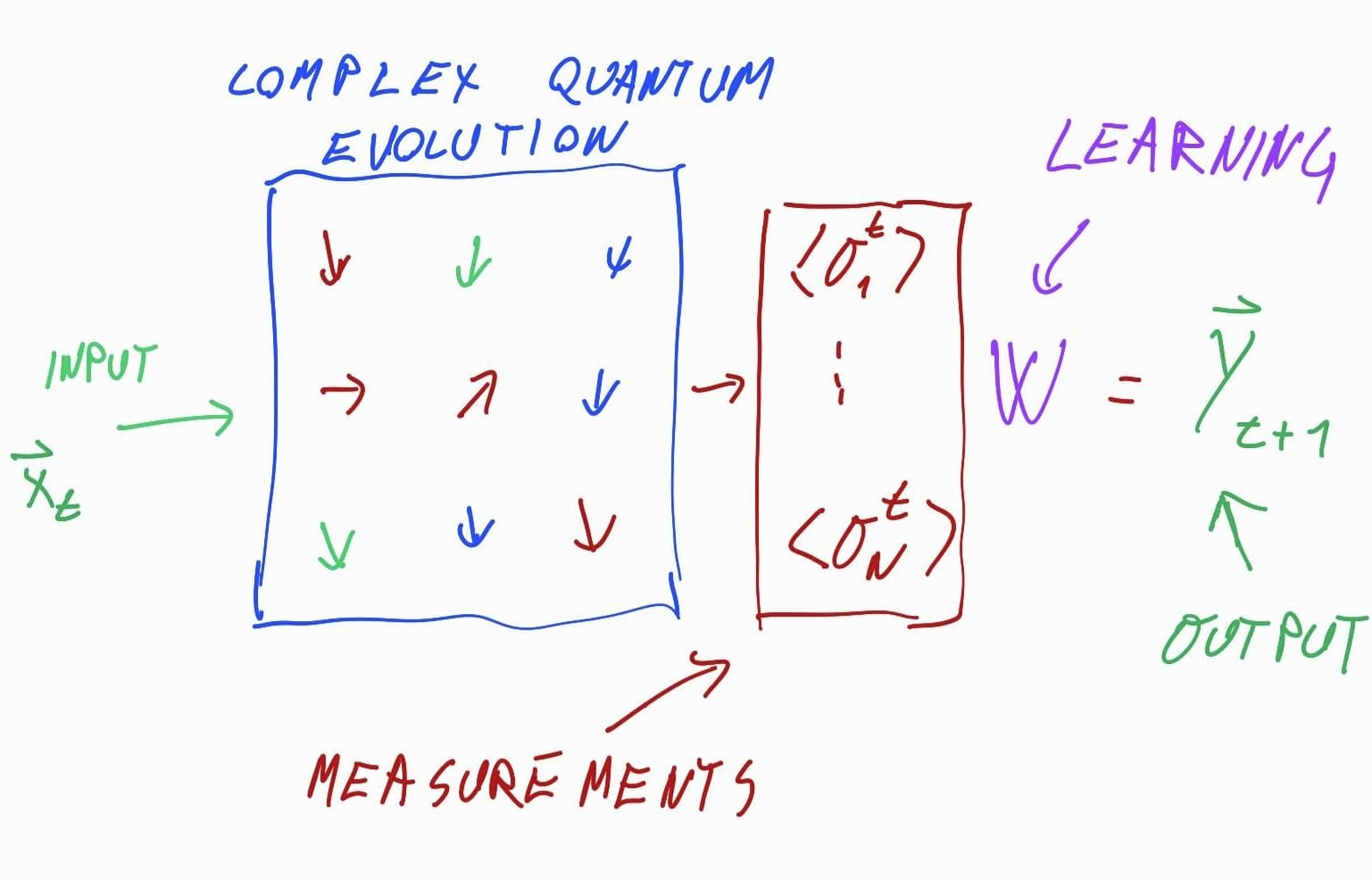
Practical quantum advantage of reservoir computing on NISQ devices -Ongoing
Training variational quantum circuits is difficult. A solution to the barren plateaus could be quantum reservoir computing (QRC), which requires minimal training or precise control, making it promising for practical quantum advantage. This project explores QRC on NISQ devices through experiments, theory, and simulations: (i) Conducting proof-of-concept experiments with muons and cold atoms. (ii) Investigating connections between reservoir computing and quantum computing. (iii) Simulating various QRC models to evaluate their utility. By integrating these approaches, we aim to assess the potential of QRC for achieving practical quantum advantage.
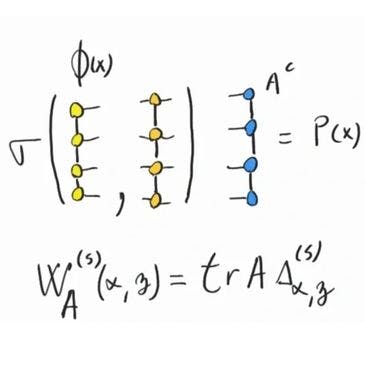
From classical to quantum machine learning through tensor networks -Finished
Machine learning relies on large amounts of data and requires significant computational power. Quantum computing, on the other hand, offers exponential speedups for certain classical algorithms. Combining the strengths of both fields is therefore promising for tackling complex problems in industry and fundamental research. This project has three goals: (i) Applying machine learning to describe quantum many-body systems, (ii) Leveraging quantum mechanics methods for machine learning challenges, (iii) Integrating quantum mechanics and machine learning to discover new applications for quantum devices.