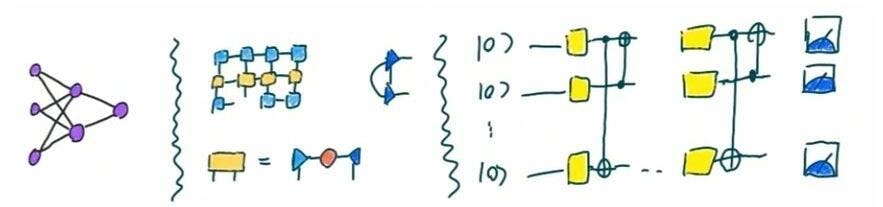
From classical to quantum machine learning with tensor networks
RESEARCH DIRECTIONS
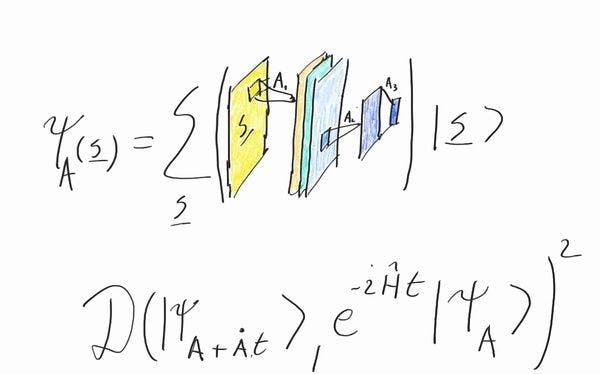
Machine learning for quantum
The first goal is to use machine learning methods for the description of many-body quantum systems. In particular, we will study transport phenomena in low dimensional many-body quantum systems with new tools that are emerging by adopting neural networks to quantum mechanical problems.
Tensor networks for machine learning
The second goal is to use methods from many-body quantum mechanics to describe machine learning problems. We will address the problems of adversarial examples, uncertainty, and generalization from a new perspective, which is motivated by the success of tensor networks for a description of many-body quantum systems.
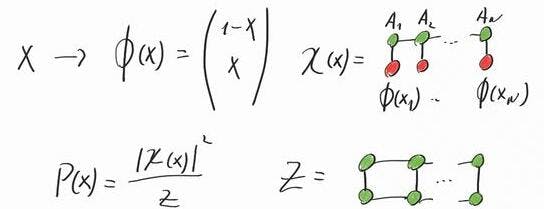
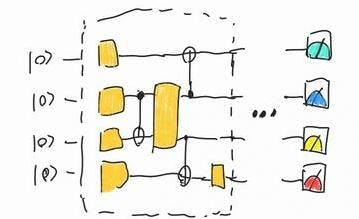
Machine learning on NISQ devices
The third and most ambitious goal is to combine the knowledge from quantum mechanics and machine learning to find novel applications of noisy intermediate-scale quantum devices with significant benefits (speedups, robustness,...) with respect to the classical algorithms. We will apply a combination of successful quantum-mechanical tools and advanced machine learning tools to find useful quantum algorithms that could demonstrate applied quantum advantage.