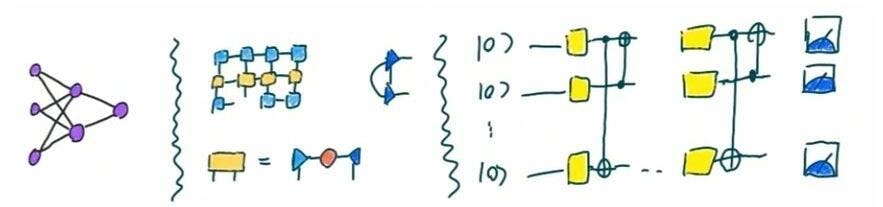
Quantum algorithms, Machine Learning, Tensor Networks
RESEARCH DIRECTIONS
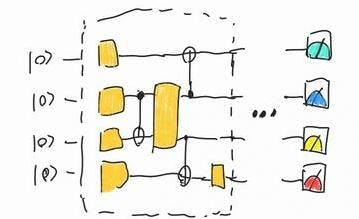
Quantum Algorithms
We leverage quantum mechanics and machine learning to discover quantum and hybrid algorithms that outperform classical ones in speed, robustness, and more. By combining proven quantum tools with advanced machine learning techniques, we aim to develop practical algorithms showcasing applied quantum advantage.
Machine Learning
We apply machine learning to describe many-body quantum systems, focusing on non-equilibrium phenomena in low-dimensional systems. By integrating machine learning methods into our workflow, we develop and explore innovative tools to tackle difficult open problems.
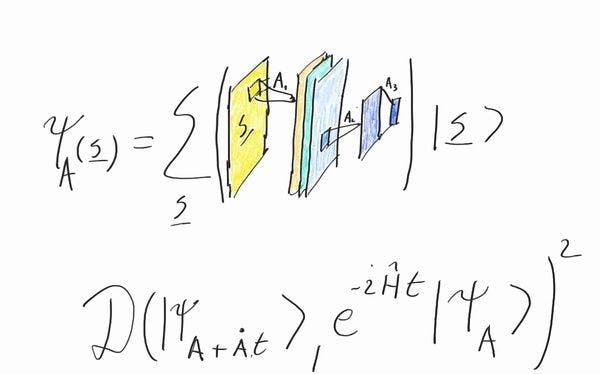
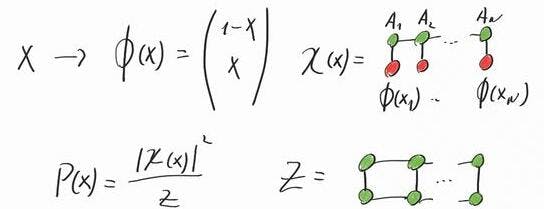
Tensor Networks
We harness methods from many-body quantum mechanics to tackle machine learning challenges, including adversarial examples, uncertainty, and generalization. Inspired by the success of tensor networks in describing many-body quantum systems, we approach these problems from a fresh, physics-driven perspective.